AI testing and development have approached a period of rapid pace, quickly impacting most aspects of human life. Whether it’s picking up books to read from an automated recommendation system on a popular reviewing platform or mechanizing complex mathematical problems, machine learning has gradually made its presence and utility felt across the board. While this trend continues, education—an aspect crucial to the development of future human generations—will also become progressively integrated with automated systems. The degree of both reliance and support, however, must be closely gauged and monitored by both current and future dispensations to ensure education does not lose its innately organic character. Regardless, an aspect of education that AI is capable of influencing considerably is testing.
Assessing students on their capabilities has been a part of human education right from the ancient periods. As education undergoes rapid modernization, the automation of assessment could be a likely possibility. Despite the potential advantages of making educational testing more pointed and accurate, legitimate concerns surrounding the overutilization of automated systems exist in academic circles. Though a more basic version of testing AI already exists in the case of OMR grading systems that check for answers on an average OMR sheet, modern AI testing methodologies show promise for expanding into further aspects of assessments and examinations. Since these developments are bound to have numerous implications on both testing and learning, an exploration of these possibilities is integral. This article considers the concerns, challenges, and possibilities of including AI testing tools in schools and universities.
Core Concerns Regarding AI Testing Tools
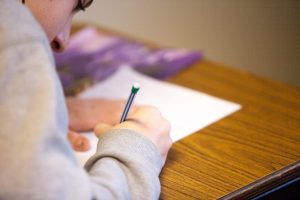
Students with unconventional approaches might be graded unfairly by AI systems.
Apart from the process of grading papers and assessing students on core abilities, there exists the potential for AI testing tools to also design and structure tests and assignments for students in the future. However, this poses a major risk and can lead to the overall depersonalization of testing. Each test is curated by teachers that have had the experiential knowledge of having dealt with a range of students with a variety of learning capabilities and methods of understanding. Deploying a machine learning protocol to structure a test for students of varied backgrounds and capacities might end up imposing a rather arbitrary process on all students. This process will also leave out crucial nuances of student learning that might be integral to their understanding of core concepts in the curriculum.
Aside from structuring tests, AI in testing also rakes up concerns surrounding assessments and evaluations. Much like the rather rigid process of instituting a one-size-fits-all approach in creating test papers, AI can also do the same when grading student performances. While this would work in the case of tests that require objective answers, subjects that entail a more individualized approach to understanding its core concepts stand at a disadvantage. AI’s straightforward approach to assessments might not sit well with disciplines that rely on a more subjective understanding to address the content and topics entailed in an assessment. The imposition of AI assessments might also lead to the curtailment of students’ creative approaches to answering questions on tests, along with hindering their ability to think critically. Outcomes of such testing and assessments could be potentially damaging to overall academic development and instead promote the devolution of learning to mere rote memorization.
The Challenges of Implementing AI Testing
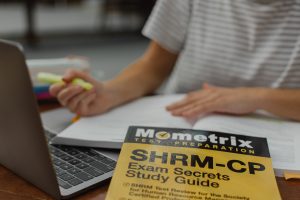
Integrating AI into the testing system will require the resolution of numerous roadblocks and challenges.
While there exist numerous concerns surrounding the potential involvement of AI in testing and assessment in academics, current theorizations in this regard might also face numerous challenges. Teachers witnessed the usage of language model AIs such as ChatGPT by students to complete their assignments in the recent past; however, deploying an AI to analyze a diverse set of student transcripts might be more challenging than just entering a prompt into a language model-trained machine learning protocol. AI, in general, still remains in a stage where there exists considerable room for improvement. Despite the impressive capabilities, consistency in results is still dubious. The complexity of AI and the data used for training and modeling are incredibly convoluted. Without a comprehensive understanding of these aspects, teachers might be unable to decode on what basis the AI carries out assessments, alongside the parameters considered for grading and analyzing student responses. The absence of clarity on these aspects will no doubt hinder the integration of AI in testing.
Another concern regarding the implementation of such protocols involves the students that might not use conventional study methods to approach learning. Their modes of expression might not fit into the generic constructs of language as exhibited by major portions of data sets on which machine learning algorithms train. Answers written by such students might be unfairly graded, impacting their academic outcomes. While this can be managed by expanding the scope of training for testing AIs, it might still be difficult to predict how the system might respond to such forms of expression even following these steps. Due to this factor, bias remains a major challenge posed by AI, something its developers work hard to weed out. Unless implicit and explicit biases exhibited by AI systems are completely addressed, the adoption of such systems remains mostly unsuitable for a task as sensitive as assessments in education.
Future Possibilities
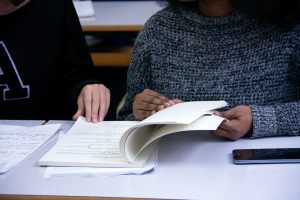
The future of AI in testing relies on how well systems are augmented to reduce bias and enhance reliability.
Despite the concerns and challenges, AI does hold a fair amount of promise when it comes to a few aspects of testing and learning in education. AI is capable of producing instant results for tests that are primarily fact-based assessments, alongside providing valuable insights to students on their capabilities. Another important future application of AI is its use in detecting cheating behavior that threatens academic integrity in a testing environment. With sufficient augmentation and training, language model AIs can also be adapted to fit the personalized requirements of students and model tests based on past performances to assess students’ weak links in the respective topics of concern. While possibilities abound about AI and its future in testing, a fool-proof, transparent, and easy-to-operate system with sufficient training provided to teachers will be the ideal method to integrate artificial intelligence into mainstream student assessments.