As ChatGPT and OpenAI’s successor language models like GPT-4 continue to rake in more users by the day, the company’s image generator AI—Dall-E—has also made a considerable mark in the industry. Competing with other major players in the field of AI image generation like Midjourney and Stability AI’s Stable Diffusion, Dall-E has garnered critical acclaim in the highly competitive market of image generator AIs. The model predominantly uses several deep learning protocols and algorithms alongside a modified GPT-3 language model tailored to image generation use cases. Currently, in its second iteration, Dall-E 2 was opened to the public around early mid-2022, whereas its predecessor was introduced in the first month of 2021. Since then, Dall-E has garnered a considerable following among AI enthusiasts, developers, and digital creators alike. While primarily known for its surreal results and unique responses to user prompts, Dall-E has come a long way in garnering a dedicated user base committed to better the outputs of the image generation model.
Like other language model AIs, Dall-E’s model, too, seems to adapt to user instructions and prompts over time, enhancing its outputs and results. The amount of detail and depth mentioned in the prompts also plays into the degree of efficiency, as less-detailed prompts are more likely to throw up absurd results with unnatural details contained in them. Consistent advances in deep learning algorithms such as Dall-E hold numerous implications for AI-generated content and subsequent human exposure and consumption of such media. The remaining sections encompass the key features and details of Dall-E.
Pioneering AI Image Generation: Dall-E’s Key Features and Prospects
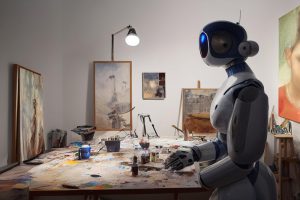
Dall-E’s creation has resulted in the development and deployment of several useful AI and ML models.
Dall-E was conceptualized and announced by OpenAI in early 2021. The name of the tool was derived by blending the names of the great artist Salvador Dali and Pixar’s famed animated movie Wall-E. Dall-E AI was designed by combining Generative Adversarial Networks (GANs) and a modified GPT-3 iteration. In the former, two distinct neural networks compete against each other in making predictions based on their autonomous learning exercises and functioning. A unique machine learning protocol, GANs have worked efficiently with language models like GPT-3 to produce impressive results in the case of Dall-E and its successor iterations. Based on the process of diffusion like other image generator AIs, Dall-E, too, converts word-based prompts to numerical vectors for the processing unit. Upon creating a vague representation of the user’s prompt, Dall-E’s processors will repeatedly refine and add more relevant features to the image based on the original description. Realism is progressively added to the image to make it more presentable. Upon the completion of refinements, the GANs work to analyze the various iterations of the image before the best results are displayed to the user.
As growing competition pits various companies and even countries as rivals in the field of the generative AI space, image generation is slowly making its mark in what is currently a predominantly chatbot-based AI market. Dall-E’s images and outputs have numerous implications for several industries. Apart from the obvious use cases in advertising and marketing, image generation protocols can also have a considerable impact on education and the increasingly digitized approach to learning. With potential visual solutions to adaptive learning protocols and classroom AI algorithms, Dall-E and its successor can also be deployed in more crucial industries to generate initial drafts of key designs and conceptual renditions. The amalgamated model allows for a great degree of flexibility and can be used in a variety of different applications.
Technical Features of the Dall-E Generator
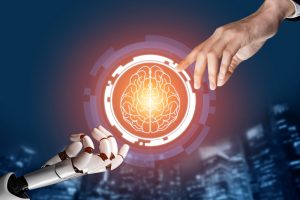
Dall-E works on a combination of NLP, GANs, and unique iterations of the GPT-3 language model.
Apart from the key role that Generative Adversarial Networks play in Dall-E, natural language processing is a key component too, where LLMs are essentially the primary mode of communication between the processors and their human users. Unlike the conventional GPT-3 model that uses nearly 175 billion parameters to power the older versions of ChatGPT, Dall-E’s model uses a truncated set, which is limited to only 12 billion parameters instead. The primary use case for this lean version is image generation, as opposed to Dall-E’s generalized language and communication-focused chatbot cousin. However, like its chatbot counterpart, a transformer neural network (alternatively, a generative transformer) also finds critical roles to fulfill in Dall-E and its successor versions.
The premise of Dall-E centered around models capable of generating entirely new and unique images from concepts and tenets of referenced objects from their training data set. These technologies will be interesting to study, as humans progress toward more advanced AI visualization and might also be integral to the development of the proverbial artificial general intelligence protocols in the future. OpenAI in an attempt to evaluate Dall-E’s generative AI capabilities also created an alternative algorithm named Contrastive Language-Image Pre-Training model, or CLIP. The firm used CLIP’s capabilities to evaluate the quality of Dall-E’s outputs and to understand the nature of the prompts which produced the best results. As Dall-E’s training was enhanced, the quality of outputs got better, eventually culminating in the development of its successor—Dall-E 2. The new iteration is now capable of delving into newer frontiers like photorealistic images and deploys diffusion techniques to accurately gain insights from the CLIP model. Dall-E is fast, accessible, easy to operate, and also highly customizable based on user choices.
The Future of OpenAI’s Image Generator
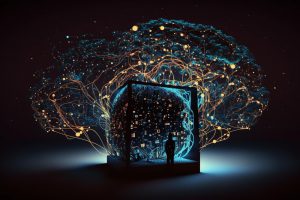
Advances in AI image generation can have a domino effect on several related domains.
Dall-E continues to rake in several users from diverse industries and backgrounds. Apart from the obvious advantages it offers to people looking to visualize concepts, its results can also inspire creative expression and can be used by creators to generate unique ideas and perspectives in their works. While creativity remains an innately human trait, the results produced by Dall-E and other advanced image-generation protocols have been beyond impressive. Though current iterations of the protocol and the general trend of AI image generators are novel, further research and development might help creators come up with more pointed use cases for these tools. Educators, too, can put these models to use whenever necessary to demonstrate key concepts and visual representations. As AI image generation progresses, Dall-E and other protocols are set to grow more utilitarian and far-reaching in their implications for society.
FAQs
1. Is Dall-E free to use?
Dall-E 2 is free for trial users. However, people looking to use it consistently will have to purchase credits that cost between $15 and $115. Each credit offers the ability to generate four images.
2. Does Dall-E 2 use GPT?
Dall-E 2 uses a modified version of the GPT-3 language model. GPT-3 works in tandem with Generative Adversarial Networks (GANs) that support the modified model to synthesize the best possible outcomes based on user prompts.
3. What are the usage rights of images generated on Dall-E?
All users are free to use the images they generate using Dall-E. The rights even include use cases such as selling, reprinting, and creating merchandise with the generated images.