Among the key disciplines in education lie healthcare and medical training. Much like other aspects of modern human civilization, AI has impacted medicine and healthcare too. Given that this sector bears with it an inseparable human component, it is common for people to think that healthcare will remain untouched by artificial intelligence and machine learning. Though the notion is partially true, it is important to understand that AI has already been making its way into the medical sector and medical education techniques. ChatGPT cleared the United States Medical Licensing Examination (USMLE), indicating that language models are indeed capable of coming up with accurate responses to questions surrounding human health and disease, at least to a degree. As growing numbers of chatbots and learnings from these tools percolate into other AI technologies, it is only obvious to expect that AI in medicine is bound to extend its influence. Similarly, as this trend continues, AI in medical education, too, will witness a further rise.
Modern medical and healthcare education is known for its rigorous nature, where students spend extensive hours studying and memorizing facts. As AI and machine learning in education become more mainstream, technology might be able to remove cognitive stresses on med school students and practicing physicians to further enhance their decision-making capabilities. The current pattern of feedback-centric generative AIs is bound to make contributions even to the healthcare education framework, allowing medical educators to assess and streamline key features of the current curriculum. The below sections take a look at the potential for AI in healthcare courses and what to expect.
AI in Medicine: Absurdity or Fine-Tuning a Key Discipline?
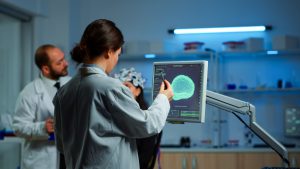
AI in healthcare education can acquaint students with a data-focused approach to their future professions.
Though the concept of AI and its use in medicine might seem novel, the first FDA-approved AI-supported medical device made its way to the market in 2016. This was followed by many other algorithms and contraptions that received approval from the governing body. AI in medicine is no new concept and the current rethink is triggered by the recent success of chatbots and language model artificial intelligence. But what can these tools do to enhance human capabilities when it comes to medicine and healthcare? While more complex iterations of AI are still going through their phases of development, simulation learning is an area where AI-supported augmented and virtual reality has already made a mark in medical education. Simulated scenarios allow students to acquaint themselves with situations that might mimic real-life doctor-patient interactions, surgical settings, and more. Not only does this allow them to test their critical thinking capabilities, but also aids in a self-assessment of their knowledge-based skills.
Medical education is also known for the density of information and the expectation from students to be proficient in key concepts and their application in practice. Adaptive learning can reduce the physical burden on students to keep preparing notes and flashcards to memorize voluminous information for each subject. Analytics and big data tools customized to fit student needs might even allow medical and allied health students to slowly adapt to the data-oriented nature of the healthcare profession. Modern healthcare relies on generating and interpreting vast amounts of patient data to provide pointed care; these tools might not only help in training students but also help manage the ever-growing extent of electronic health records. Moreover, intuitive AI tools might open up possibilities for more streamlined data collection methods that will be essential in carrying out population-based medical studies and research.
Challenges in the Application of AI and Machine Learning in Healthcare
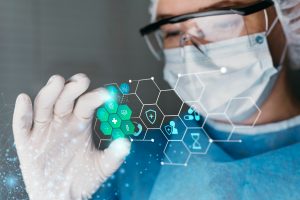
The medical sciences are sensitive subjects and must be dealt with carefully while integrating AI systems.
Like the other applications of AI and ML, their usage in medical education, too, has a range of ethical concerns and challenges. Among the most concerning are issues arising from AI bias. Training data sets and algorithms are shaped out of defined blocks of data. Artificial intelligence and machine learning models can sometimes become unreliable in situations where information out of these data blocks’ extent is involved. Moreover, human bias encoded within the training data, alongside faulty extrapolation, can also have a significant impact on judgment making. These issues take on a different form and cause problems that are now referred to as AI hallucinations. Such concerns might spell serious issues for AI in medical education and mainstream healthcare operations. Transparency and security are other concerns, since healthcare is a field that works with voluminous amounts of personal information and data. Algorithmic models often end up relying on experiential learning, and not all stakeholders will be amenable to their personal information being used to improve AI models. Security risks also expose artificial intelligence systems to data breaches and privacy concerns.
Given that healthcare is a rather sensitive discipline, educating students with the right approach is crucial. Potential errors arising from AI or machine learning in healthcare operations also open up a new world of concerns that relate to liability and accountability. While medical negligence and malpractice laws are fairly straightforward in the current world, the introduction of AI and ML in this regard might complicate matters, forcing a reevaluation of extant norms. Lastly, artificial intelligence does not possess intuitive thought, and it will have to be their human operators that will still bear responsibility for judgment. Regardless of current attempts to develop responsible artificial intelligence, human supervision will be irreplaceable when it comes to AI in medical education.
Medical AI and Future Developments
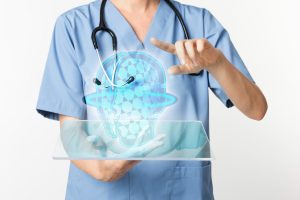
Data science can transform the way healthcare is understood and practiced.
AI in healthcare has tremendous potential to improve patient outcomes and the efficiency of healthcare professionals. Integrating these technologies right during the training period for these individuals might just provide them with much-needed education on handling and operating these tools in their careers. As technologies like intelligent tutors supported by AI-generated content creates an impression on medical education, medicine is bound to become a profession that utilizes data more efficiently and intelligently. That being said, crucial integrations with mainstream medical frameworks are still a few ways down the road. Careful examination of these tools along with sufficient trial runs will be needed to impartially assess what they can offer to trainee medical professionals and students. Achieving the middle ground will include the integration of intuitive human judgment with the mechanical efficiency of artificial intelligence to achieve diagnostic accuracy, treatment effectiveness, and surgical success.