Artificial intelligence and machine learning have evolved to produce intelligent systems that are capable of identifying patterns in vast volumes of data and information. Academic research often yields statistics that can extend into quantities beyond human capabilities. AI for research has been gaining popularity since computing systems and trained models can automate the process leaving behind minimal scope for error when primed effectively. Moreover, AI in research might also help with the collection and segregation of crucial information, which when done in the initial stages of studies can greatly promote ease of sorting through volumes of data. Apart from these obvious use cases, language model AIs have also been used to generate research papers on topics, leading to concerns regarding ethics and the retention of quality academics in the face of rapidly evolving AI technologies.
Artificial intelligence’s research areas have found an important implication in data science, a discipline that is slowly helping transform other areas of research. Better predictive models can allow researchers to study portions of information that might be only barely noticeable in the background of all the other information collected from a particular study. With AI already improving its capabilities to generate images and identify them, artificial intelligence is no doubt being further developed to identify even real-world patterns apart from abstract and mathematical data sets. That being said, the role of AI in research and the potential it holds for future researchers requires careful attention since disciplines that determine the future of humanity cannot be blatantly automated without assessing AI in these roles from a neutral standpoint.
AI for Research: Current Trends and Perspectives
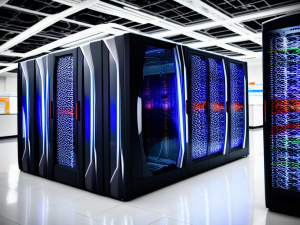
Advanced computing systems can have a major effect on the way researchers analyze their data.
Academic research drives forth the quest for knowledge and also allows academicians and researchers to identify priorities in knowledge acquisition. Moreover, research is key when it comes to detecting and determining gaps in knowledge to better address areas of various disciplines that might require further studies to reinforce. The results of research directly impact real-world outcomes either via influencing various curricula or leading to the creation of tangible systems and inventions that aid the progression of humanity and its knowledge. Introducing AI in research, while intriguing at first glance, might also involve an additional layer of complexity. While it remains obvious that research-centric AI can allow academics to let go of their cognitive load and focus on the more crucial aspects of research, it also becomes apparent that the introduction of AI in research processes also poses risks to innately human roles in these systems, one of them being intuition. Since artificial systems cannot be trained to think intuitively, a human role will remain indispensable to the nature and function of academic research.
Despite the expected rise in productivity and efficiency, AI research might end up presenting unique challenges such as the introduction of bias—a factor researchers work hard to avoid and weed out from their research methodology. Apart from these concerns, research in the academic setting is also defined by the establishment of set roles and individual contributions; introducing an expansive generative artificial intelligence in the mix might just dilute distinctions between these roles, leading to increased confusion and potential replacement of the human element in research. The role that academic research plays in the furthering of economic development is crucial, and the production of quality research is integral to the R&D sectors of countries. With the rise of chatbots such as ChatGPT, even structuring and writing research papers has become possible through language model-supported artificial intelligence and AI research paper generators. This raises additional concerns and questions surrounding the role of AI in research and writing since the communication of data retains centrality in an academic environment.
Research AI: The Potential to Do Good
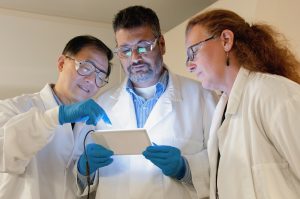
AI can simplify certain areas of research, helping improve both the quality and productivity.
AI in research, like in other disciplines, is not without problems; however, explorations into the niche came into being to support researchers and academicians make better use of their time alongside maximizing the efficiency and quality of research. Despite there being several concerns associated with these aspects of research AI, there still remains enough potential for artificial intelligence to benefit knowledge acquisition. Some of these potential benefits are listed below.
1. Gap Identification
AI has the potential to determine missing chunks of information and data in research papers and statistics. Natural language processing and advancements in data science can allow researchers to deploy AI in identifying faulty data sets while also determining areas that require more accurate information to support their endeavors.
2. Detection of Data Tampering
Artificial intelligence in research can aid reviewers and governing authorities to identify data points that might have been augmented to arrive at a desired research outcome. This will help research bodies weed out subpar and manipulated research articles in favor of better ones that are more suited to the enhancement of their respective disciplines.
3. Identification of Funding Sources
With voluminous amounts of research and results being produced every day, it might make it difficult for both researchers and funding partners to identify research outcomes that fit their requirements. AI can help sift through vast sets of research outputs to help researchers find sponsors and fund their efforts.
4. Quality Control
AI in research can aid with the enforcement of qualitative guidelines for researchers and their papers. Manual monitoring can often be quite time-consuming and the utilization of artificial intelligence in this regard can speed up the process of improving the quality of research and its reporting.
The Future of AI Research
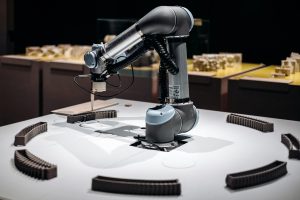
The future of research relies on upscaling both skill and quality of data.
Though there still remains a considerable degree of debate surrounding the role of AI in research, simplifying the process of research and reducing the cognitive load on researchers remains a core concern that will invariably require a degree of computing assistance. The propagation of responsible AI and artificial intelligence structured around the basic principles of remaining adherent to core ethical principles will remain crucial to the use of AI in research. From a perspective that provides primacy to productivity and the build-up of epistemic wealth, the acquisition of AI assistance in the field of research seems rather inevitable. However, paying close attention to the nature of its functioning in research environments and identifying key patterns will determine the future of automated systems in both field studies and data laboratories.