We live in an era where a renewed push to create intelligent machines has finally borne fruit after decades of effort. Clearly, the current dichotomy between machine learning and human intelligence is something that humanity might have to address and reconcile. While it is known that machine learning and AI are both based on the tenets of human cognition, anyone with a basic understanding of modern computing is aware of the differences. Despite the numerous advancements in the creation of neural networks and the more recent successes of language model AIs, humans have so far been unable to recreate human intelligence in the laboratory. Machine learning algorithms, despite their inherent purpose of supporting human efforts and cognition, have sparked fears of replacing several jobs through automation. Though the inherent fallacies as well as truths in these assumptions are part of a separate discussion, leveraging both synthetic and organic intelligence might not only be good for humans but will also benefit the overall development of efficient machine learning protocols.
With the advent of the information revolution, the rate at which civilization began amassing data quickly outpaced the capacity of the average human being. To support the constant rise and speed with which humanity produced information, the development of intelligent machines seemed inevitable. In the aftermath, disciplines like analytics and data sciences evolved to support quick and coherent processing. Evidently, humans are at a stage where synthetic intelligence is not only an accessory but also a necessity for the smooth functioning of civil society. Bearing this in mind, the article explores the importance of bringing together both human intelligence and machine learning to exemplify the necessity of both.
Human Intelligence vs. AI and Machine Learning
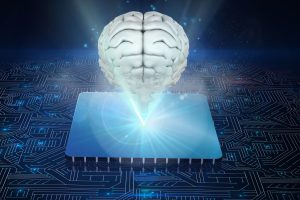
Current AI protocols, despite their excellence, are no match for human intelligence.
Delineating the differences between human intelligence and AI is crucial to identify where and how these two can complement each other. Despite the inherent differences, the prowess of human intellect and capability made it possible for civilization to build intelligent machines. Moreover, as one explores the depth of artificial intelligence, a clear pattern emerges and hints at all intelligent machines having been based on models that aimed to mimic the human brain. That being said, artificial intelligence and machine learning constitute a set of autonomous protocols capable of carrying out certain tasks with little to no human interference in the process. Most of these computational processes commonly include mathematical functions, language decryption, big data, analysis, and extrapolation. A good portion of these tasks is a combination of multiple machine learning algorithms that work in tandem to achieve increasing levels of accuracy and efficiency.
As opposed to machine learning, human intelligence is a phenomenon that has arisen through evolution. Human intelligence also seems to vary depending on factors such as nutrition, environmental factors, emotional stressors, and overall education. Characteristics like emotional processing capabilities, situational awareness, creativity, and rational thought arise primarily as a result of human intelligence. Crucial human tendencies like intuitive thought and critical thinking are also inseparably linked to the notion of intelligence in human beings and are often the key distinguishing factor that draws the line between natural and synthetic cognition. The human mind is no match for its mechanical counterpart in domains such as abstract reasoning, speed of thought, and in the notion of personalized memories. However, it is also apparent at this point that machine learning has become integral to human functioning merely due to the complexity of modern human society and the systemic juggernauts it has created to aid its expansion into various untapped domains of the natural and artificial sciences. Offsetting each of their weaknesses with a blend of both might just be the middle ground necessary for sustainable development.
Human-in-the-Loop Machine Learning: A Potential Solution
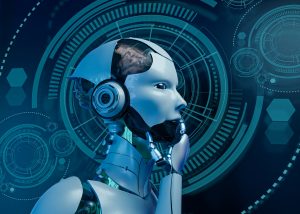
Human-in-the-loop machine learning can be a viable solution for existing challenges in AI.
All machine learning algorithms invariably require human intervention to achieve acceptable degrees of functionality and accuracy; however, recent advancements such as generative AI models like GPT-4 and others have also witnessed considerable degrees of unsupervised learning in AI. Though all AI and ML algorithms rely on data generated by human effort, advanced systems are increasingly dependent on autonomous training and learning structures to take the weight off their human designers to reduce the strain involved in optimizing them. Despite these independent learning curves, humans are often required to step in when these machines encounter problems they cannot solve. This is where feedback becomes crucial. It comes as no surprise that machines are capable of making mistakes, just like their human creators. Machine learning algorithms rely on statistical analyses and data to arrive at judgments. Regardless, these judgments can be flawed and lead to erroneous conclusions. Human feedback in such instances comes as a boon for the development of intelligent machines as it allows them to modify their approach instead of sticking to their data set as absolute. Machine learning models constantly alter their approach based on new information and feedback from human operators. This is essentially a human-in-the-loop model that allows for the presence of organic intelligence in the learning curve of the algorithm.
Although, human-in-the-loop models are not merely restricted to mere feedback loops either. This approach essentially tries to bridge human and mechanical capabilities to help solve problems that are beyond the scope of both. With the human-assisted learning curve, machine learning protocols are fed labeled datasets to understand the overall performance of the algorithm. While autonomous learning does allow machines to discover patterns in vast and unstructured data, human-in-the-loop models can act as a failsafe against AI bias and even prevent and reduce the prevalence of hallucinations. It also makes sense to have humans in crucial stages of ML development, since these systems will essentially cater to the requirements of other individuals and groups.
Human-in-the-Loop Models and Their Academic Prospects
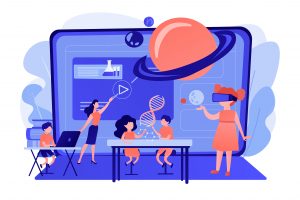
Human-in-the-loop models can be crucial for the future of AI in academia.
The development of responsible artificial intelligence has several implications for the future of AI in academics. Thus, the necessity of human intelligence and supervision in machine learning algorithms that might find applications in education cannot be overstated. As previously established, a balance between both human and artificial intelligence has the potential to create better machine learning protocols and might just help us address mutual deficiencies. The presence of human checks and balances in machine learning development also raises the level of accountability involved in the process as opposed to autonomous deep learning methods. To create systems that benefit students and shape their future, AI and ML must be defined with clear goals that meet the necessary standards. This can only be achieved with sufficient supervision and involvement in existing machine learning protocols—a balance that can be achieved with human-in-the-loop models.