The past decade, and specifically the last year, has seen a massive upsurge in demand for AI tools and chatbots. Needless to say, the potential of machine learning has skyrocketed with people being exposed to the many wonders of autonomous algorithms and their ability to respond to human queries. Add to this consistent advancements in computing and processing technologies, algorithms are becoming all the more powerful and robust in their capabilities. Currently, humans are pushing the limits of processors by compressing the size of each chip down to the atomic scale. Soon enough, scientists will run out of limits to breach, unless of course there are considerable advances in quantum computing. However, the demand for AI and powerful deep learning protocols is only growing, and building appropriate neural networks in the available scales will be a challenge for forthcoming generations of professionals. That being said, a novel type of neural network has shown a fair degree of promise in handling the steep requirements of AI models and ML algorithms.
Apart from these use cases, deep learning and data analytics go hand in hand with artificial intelligence protocols. Efficiency and simplistic operability are quintessential. Optical neural networks (ONNs)—a potentially effective alternative to conventional options—might just be the key to solving hardware challenges faced by the AI domains. ONNs essentially integrate optical components that use light as the medium of communication to transmit signals within the framework. This is cost-effective as well as energy-saving, essentially mitigating the environmental burden placed by conventional AI processors and artificial neural networks.
What is an Optical Neural Network?
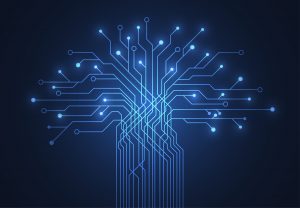
ONNs offer potent solutions to rising energy costs of maintaining deep learning models.
Artificial neural networks essentially mimic the human brain. Much like how an impulse traverses the neuron’s axon, making its way to the nerve endings before reaching the synaptic junctions that transmit the impulse to other neurons, artificial neural networks perform a similar function. In the case of optical neural networks, the signal is transmitted through electromagnetic waves (in the form of light), as opposed to electrochemical pathways in a biological setup. The earliest conceptualizations of optical neural networks date back to the 1980s when optical components were understood to be a fair replacement for conventional processing and transmission units. As opposed to using electrical signals to transmit information, optical neural networks rely on light and refractive surfaces. Such models have been found to enhance the efficiency of processing power in AI and ML algorithms. As demand for chatbots witnesses a steady rise, optical neural networks might see more implementation in neural frameworks of famed AI interfaces.
Early optical neural networks were designed to use volume holograms or holograms that depicted objects in three dimensions when transmitting data along with dedicated input and output arrays. The progress of AI research has since led to better, more efficient models that are capable of simplifying convoluted structures and offering similar or even better results in more compact paradigms. Tasks like natural language processing can benefit greatly from optical neural network integration, given that the efficiency is higher, while the costs of operation are considerably lower. Functionalities like big data, too, can be streamlined using these novel processing units. Researchers are currently working with two types of optics to enhance these neural networks. This includes silicon-based optics, which involve the creation of minute tunnels to guide the lightwaves, and free space optics. The latter uses air for propagation. The former has better speed, while free space optics offers better parallel communication. Scientists are leveraging both systems to decipher the ideal options for optical neural networks.
Deep Learning vs. Machine Learning: How Optical Neural Networks Can Help
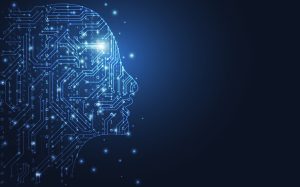
Deep learning is bound to require faster and more efficient neural networks in the future.
While machine learning is the overarching discipline of training algorithms on data to detect specific patterns and to carry out autonomous computations, deep learning entails a highly specific subset of machine learning. Deep learning protocols involve the extensive usage of artificial neural networks to enhance the performance of machine learning models. By mimicking biological neural structures, artificial neural networks can enhance the degree of autonomous learning and the performance of machine learning algorithms. Major chatbots such as ChatGPT, Bard, and Claude all rely on deep learning protocols that support their underlying large language models. These models essentially function as if they have some rudimentary level of intelligence, being capable of carrying out executions of programs and addressing problems independently. The growth of demand for language model chatbots is only bound to increase, and promoting efficient functioning will be a key point on the agenda for future neural networks.
Optical neural networks and deep learning have a growing relationship. If optimized, these novel approaches to AI computing can provide better speed and efficiency of output to deep learning systems. ONNs perform the most complex computations in neural frameworks with better energy efficiency as well. The skyrocketing energy costs imposed by deep learning and the following environmental impact have been a cause for considerable concern among both developers and policymakers. Optical neural networks offer a viable solution to this problem. Other advantages of ONNs include vast storage capacities coupled with the ability to perform several complex computations simultaneously. Lastly, optical neural networks can enhance the learning ability of the framework without adding to the complexity of the existing architectures.
The Future of Deep Learning and ONNs
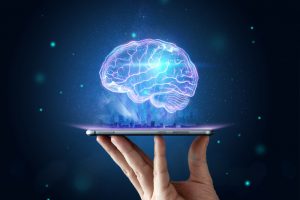
Research into cost effective optical neural networks is bound to impact deep learning in a major way.
Optical neural network research is actively ongoing, with the potential to determine the future trajectory of deep learning as a whole. The field is constantly evolving, with new learnings being reported regularly. Scientists have moved from volume holograms to more advanced transmission techniques that have drastically improved the extent of computation and processing power. Apart from this, integrating ONNs with photonic chips is also an area of active exploration. If humans intend on creating elusive technologies such as artificial general intelligence, prioritizing energy efficiency, computational speed, and effectiveness will be integral. Optical neural networks address all of these requirements and more. Future developments in these technologies will hold important implications for the AI and ML domain.
FAQs
1. Why are optical neural networks faster than conventional ones?
The utilization of light for communication allows for parallel processing and computation, making ONNs faster as well as energy efficient.
2. Are optical neural networks expensive?
The initial cost of implementing optical neural networks seems to be on the higher side. However, this is mitigated by its ability to consume lesser power, making it a good investment in the long run.
3. What are the challenges to optical neural networks?
Complexity and standardization issues are the key challenges faced by optical neural networks. High initial costs are also a complexity faced by developers and researchers looking into ONNs.